COVID-19 has presented to the world many unprecedented challenges, the most prominent of which are the health and economic crises that are currently unfolding.
While Central and state governments in India are struggling and working under immense pressure to respond to these twin challenges, there is a third related challenge that is now the elephant in the room – data.
Governments and most government-owned organisations have been known to operate mainly through physical files. In the early 2000s, this gave way to the IT revolution. Governments went online as the idea of ‘e-government’ became popularised as a magic bullet to the red tape of the physical file. A new website was launched every few months, but at the backend operations to update and maintain the ‘open data platforms’ have remained largely manual. The pandemic and its consequent crisis have uncovered and highlighted this issue more than anything else in the past.
Governments are now required to update, track, monitor, analyse and present data real-time, and all this with manpower that is still used to manual data entry and report preparation. As a result, there are inconsistencies and contentions to reported numbers that reach the media. The biggest challenge, for example, with the migrant labour issue was not the provision of services and benefits. The issue was that no government (Centre or state) had a count of how many citizens had actually migrated, whether in the state of origin or destination. Consequently, quick and impulsive steps taken by Governments were able to help many and yet exclude large number of potential beneficiaries, as has been highlighted by multiple media reports and news channels over the last month. This is not to say that the governments didn’t do enough. Most state governments put in humongous amounts of resources and efforts to try and reach every eligible beneficiary but faced an acute shortage of information on their numbers, location and requirements.
Another example of data mismanagement has been recently seen with the inconsistency between the number of hospital beds reported over the mobile application in Delhi and that confirmed by hospitals to citizens.
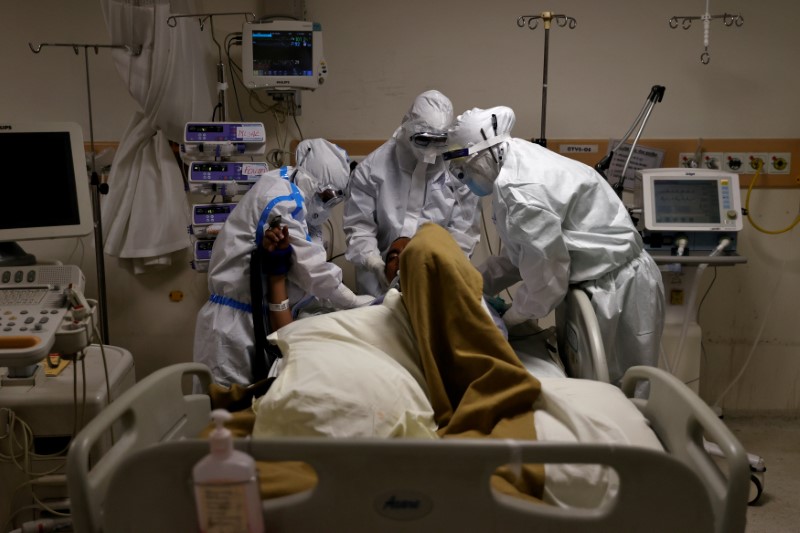
Medical workers wearing take care of a patient suffering from COVID-19 in New Delhi. Photo: Reuters/Danish Siddiqui
When it comes to managing COVID-19, the urgency to collect and maintain databases is even more acute. Data is needed to understand the disease spread, contact tracing and outcomes of the disease (hospitalization, recovery, death rates). Each of these is intensely scrutinized in the public domain. Governments have had to put in record speed to build data systems. But such mission mode approaches often risk creating a bigger problem than no data.
To illustrate, the COVID-19 lifecycle for any individual has primarily 4 components – sampling, testing, treatment and outcome. The guidelines around collection and maintenance of sampling and testing data are as per the Central Government whereas the datasets around treatment and outcome fall under the states’ responsibilities. As a result, the records are maintained separately, and the states struggle to comprehensively access and then integrate the data from the Centre into the state database. The consequences of the struggle have unfolded in different ways across states but here is a taster of the kinds of problems states face. There is no single unique identifier for each patient across these databases.
Also Read: Lockdown, Shutdown, Breakdown: India’s COVID Policy Must Be Driven By Data, Not Fear
To add to the complexity, in most cases, the records under treatment and outcome are maintained by each facility independently and individually which makes cross-referencing with the Central databases even more difficult. Collective compilation of all data at the state level is a task that is being resolved even now and thus results in reports of data inconsistency and backtracking in the media. We’ve seen these inconsistencies unfold in Delhi over the number of hospital beds reported in the app vs in reports by individual hospitals.
The issue doesn’t end here. When a patient is transferred between facilities upon the development of severity in her/his condition, there is, in all likeliness, a duplicate record created instead of an update to the existing one. This adds to the challenges of cross-references and data cleaning for the purposes of compilation and analysis. Datasets on contact tracing and quarantine convolute the exercise further and between the Central and state governments, the solution does not seem to have reached an effective conclusion yet.
So, while, it is easy to pull out an indicator from any one of these datasets (such as the number of samples taken or tests done on a particular day), it would be extremely difficult, if not impossible, to compute an indicator that requires cross-referencing of patient’s data (such as the average number of days a COVID-19 patient took between the onset of symptoms and discharge/death). In order to estimate the number of patients that were admitted with mild or no symptoms but later developed a severe condition and required ventilator support, the user would need to reference records maintained in each healthcare facility and try and match unique patient records. The user may still not be successful. And all of this because when the data collection exercise began, the objective was to collect data and not to analyse it to reach a useful conclusion. Therefore, there was no thought put into a system to trace each suspected/infected individual from sampling or onset of symptoms as reported till discharge/outcome. Had the government systems – Central and states – been accustomed to data as evidence for decision making and data for better governance, the approach would have been tremendously different from the beginning.
It is worth a mention that over the past two months, most governments have realised this and have tried to make amends to the structure such that these datasets can be integrated and utilised better for decision making. While that may still fail to address the issue with legacy datasets, the new systems being built should be thought through in terms of “the objective” or “requirement for decision making” instead of data availability at each step of the process.
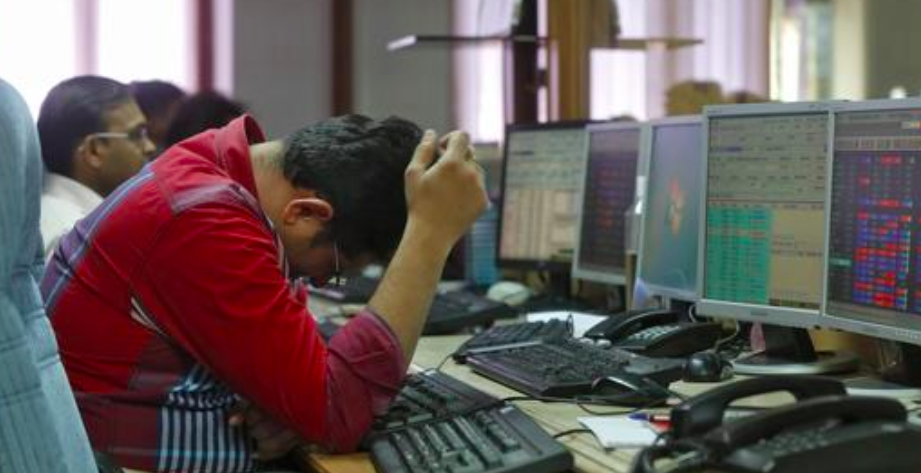
The new systems being built should be thought through in terms of “the objective” or “requirement for decision making” instead of data availability at each step of the process. Representative image. Photo: Reuters
The need of the hour is to realise that internal data management is an urgency and requires a dialogue between policymakers, experts and academics. This dialogue should identify what data are relevant, necessary and aligned to decision-making goals. There is no universal template or standardised plan of data management for a government. Each needs to create a framework for data management, analysis and usage depending upon the current frameworks and systems in each of the multiple departments that work independent of each other. Every department should be looked at independently with the flexibility to create and maintain a different solution for each. These systems should be across all levels of the hierarchy and interoperable with each other. Manpower, at all levels, should be trained and mandatorily made to use technology solutions to enter, maintain and share data.
The COVID-19 pandemic has highlighted and brought to the forefront an issue that would have otherwise lingered for years to come without focus or attention. It has made apparent that provision of services can be much simpler, more accessible, transparent and efficient with clearly defined procedures for data management and dissemination.
A checklist for governments to prevent from falling into short term unsustainable solution traps may be as below:
Before any data collection or restructuring exercise, think of the objective. Against each datapoint, ask why it is needed and which data point should be the anchor of the dataset
Do not create short term technology solutions and processes for data management. The crisis has presented an opportunity to create long term solutions. While COVID-19 data management is critical and a priority in these times, making systems that can be extended to accommodate other datasets and programmes are as crucial.
Do not get satisfied with a dashboard that presents to the administration or the people charts and graphs with everyday updates. Focus on the backend – data entry, processing, storage and analysis
Invest in teams that can help build data management capability inside the departments. Third parties that offer solutions and step back post the contractual duration may leave the internal teams with challenges instead of solutions. Push existing staff to upskill and learn digital solutions.
Schemes and programs change over time. Ensure that each solution is modular and flexible to accommodate new conditions and features.
Data privacy and security are of utmost importance. All portals and systems should comply with the protocols to secure the datasets and maintain their sanctity.
Above all, technology is only a tool that should enable transparent and efficient processes and management. It is crucial to first define the processes and guidelines, and document them and then take support from technology solutions to digitise the defined steps and guidelines.
Process re-engineering is not the same as digitisation of processes. Re-engineering should ideally result in shorter, faster and more efficient processes. Digitisation can then make these processes more accessible, transparent and accountable.
Tulika Avni Sinha is a researcher working with the Department of Governance Reforms, Government of Punjab.