The Delhi police reportedly used automated facial recognition software (AFRS) to screen the crowd during Prime Minister Modi’s election rally in Delhi last December. This was also the first time Delhi police used facial images collected across protests in Delhi to identify protesters at the rally.
New categories of deviance such as ‘habitual protesters’, and ‘rowdy elements’ have emerged as faces of protesters are matched against existing databases and maintained for future law enforcement. Police departments in a growing number of states also claim to be using facial recognition and predictive analytics to capture criminals. The Railways intends to use AFRS at stations to identify criminals, linking the AFRS systems to existing data bases such as the Criminal Tracking Network.
Also read: Is Delhi Police’s Use of Facial Recognition to Screen Protesters ‘Lawful’?
The Telangana State Election Commission is considering using AFRS to identify voters during the municipal polls in Telangana. The home ministry recently announced its intention to install the world’s largest AFRS to track and nab criminals. AFRS adds to growing list of surveillance systems already in place in India, such as NATGRID and the Central Monitoring System, even while there continues to be little publicly available information about these programs. A recent study by Comparitech, places India after China and Russia in terms of surveillance and failure to provide privacy safeguards.
Automated facial recognition systems are a direct threat to the right to privacy. Unlike CCTV cameras, they allow for the automatic tracking and identification of individuals across place and time. Footage from surveillance cameras can be easily cross-matched, and combined with different databases, to yield a 360-degree view of individuals. As facial recognition systems combine constant bulk monitoring with individual identification, anonymity is further rendered impossible – there is no protection, or safety, even in numbers.

Unlike CCTV cameras, facial recognition systems allow for automatic tracking and identification of individuals across place and time.
But much more is at stake than individual privacy.
AFRS can have a chilling effect on society, making individuals refrain from engaging in certain types of activity for fear of the perceived consequences of the activity being observed. As Daragh Murray points out, this chilling effect results in the curtailment of a far greater set of rights, such as the freedom of expression, association, and assembly. Taken together, this can undermine the very foundations of a participatory democracy.
With AFRS and other automated electronic surveillance systems, suspicion does not precede data collection, but is generated by the analysis of the data itself. To avoid suspicion, people will refrain from certain types of activity or expression; and the worry or threat, of not knowing what data is being collected or how it is being combined and analysed, can result in the self-censorship of a wide range of activities. Such surveillance, as Christian Fuchs points out, first creates a form of psychological and structural violence, which can then turn into physical violence.
Further, because surveillance operates as ‘a mechanism of social sorting’, of classifying individuals based on a set of pre-determined characteristics, and their likelihood of posing a risk to society, the chilling effect is likely to be experienced more severely by already discriminated against communities. Such social sorting is further likely to exacerbate identity politics in India, enforcing and exacerbating social divisions.
This is also why critiques of AFRS that point to their low accuracy rates or failure to identify certain skin tones miss the point entirely. A more effective system would pose an even greater threat to privacy, social sorting, and participatory democracy.
Much of the criticism around the deployment of AI-based technologies has highlighted issues of discrimination and exclusion; and how this can result in the violation of human rights. But the case of AFRS shows how AI systems can not only result in the violation or loss of rights, but are also productive of certain types of behaviour – creating a disciplinary society.
Further, because chilling effects is some sense rest on the occurrence of non-events – i.e not engaging in particular types of activities – frameworks based on identifying discrete violations of rights are likely to be inadequate. The case of AFRS thus highlights how conversations around AI governance need to move beyond the identification of immediately visible harm, at an individual level, to ask what kind of transformations are taking place at a structural level – how values of privacy, liberty, democracy and freedom are being recast.
Also read: India Is Falling Down the Facial Recognition Rabbit Hole
In India, as elsewhere, surveillance technologies have entered the public domain through a narrative of both safety and protection; and, consumer convenience and personalisation. The rhetoric of safety, for example, is behind the recent allocation of the Rs. 250 crore from the Nirbhaya fund for the installation of facial recognition cameras at 983 railway stations across the country.
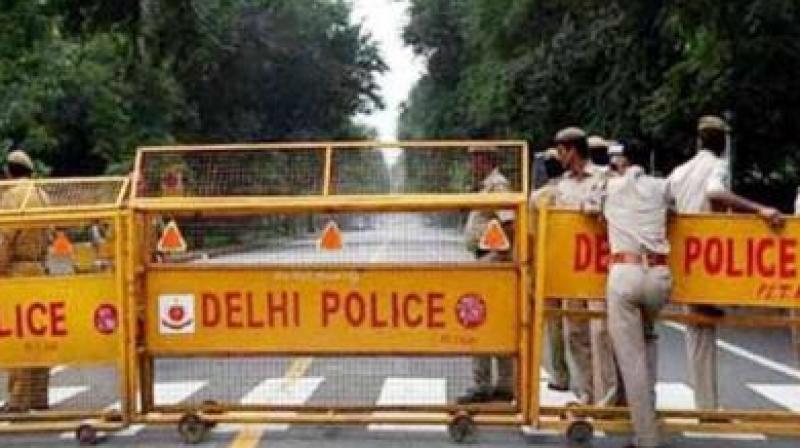
The Delhi Police has registered 10 criminal cases against those involved in rioting and arson during the Anti-CAA Protests. Photo: PTI
Automated facial recognition software earlier procured to trace missing children in the country, are now being used to sort and profile citizens dissenters and peaceful protesters. This shows the folly of searching for the good use-cases of AI. AFRS, like other surveillance techniques, are also being routinised and normalised through the promise of consumer personalisation and convenience – whether the embrace of facial recognition to unlock an iPhone or people voluntarily signing up for AFRS at airports.
Mark Andrejevic has argued, for example, that the ‘key to the creation of digital enclosures today is the emphasis that has been given to the technologies of liberation, in particular, mobile phones and social networking sites.’ This ‘domestication of the discourse of interactivity’ has been crucial for expanding the means of surveillance. As a result, as Lyon notes, references to an Orwellian dystopia are ‘rendered inadequate because of the increasing importance of nonviolent and consumerist methods of surveillance.’
With the various government ministries seeking to employ AFRS, many have called for regulating the use of AFRS – that the conditions of its use must be specified as well as the necessary judicial processes established. But, regulating its use is not enough. Even if AFRS were permitted in only a few select instances, or after due process has been followed, the chilling effect on democracy will remain.
At a more practical level, the effectiveness of AFRS requires the collection of biometric facial data from all individuals, not only the targets of surveillance, or those suspected for criminal activity. Selective use also contributes to normalisation and routinisation (and over time, even more effective AFRS). Let’s not forget that many surveillance technologies are first tested in the criminal system before they are deployed for the broader public.
Even with adequate legal safeguards, and perfectly accurate facial recognition systems, the harms to society far outweigh any possible benefits. We need to ban the use of AFRS all together – to establish this as a necessary red line to preserve the health and future of democracy. Even while effecting such political change may seem a far cry in the current political climate, it is urgent to start building at least a normative consensus within civil society.
Also read: Delhi Police Is Now Using Facial Recognition Software to Screen ‘Habitual Protestors’
This conversation has already started in other corners of the world – San Francisco has already banned the use of AFRS by the police and all municipal agencies and the EU is considering banning the technology in public spaces for five years. Neither go far enough – an even better example could be Portland, Oregon, which is considering banning the use of AFRS by both government agencies and private businesses. While India continues to lack any frameworks for the governance and regulation of AI-based technologies, the case of AFRS highlights how this is an urgent priority.
AFRS will soon be complemented by systems for emotion and gait recognition; technologies that detect heartbeat and micro-biomes are also under development. We need to act now: as these technologies become more embedded in not only governance systems but only consumer habits, there will be fewer opportunities for course correction.
Urvashi Aneja is co-founder and director of Tandem Research and tweets at @urvashi_aneja. Angelina Chamuah is a research rellow at Tandem Research.